AI in Finance is no longer a distant vision—it’s the driving force behind the transformation of modern financial markets. From dynamic portfolio management to real-time fraud detection, artificial intelligence is reshaping how both individual investors and major institutions make decisions. In 2025, AI-powered tools have become essential for staying competitive in a fast-moving, data-driven financial world.
This comprehensive guide explores the key trends defining AI in Finance, offering in-depth insights into how technologies like large language models (LLMs), predictive analytics, and autonomous trading systems are revolutionizing everything from sentiment analysis to ESG screening. Whether you’re a retail trader, fund manager, or fintech enthusiast, understanding these developments is critical. Through real-world examples and emerging innovations, we’ll break down how AI is creating smarter, faster, and more adaptive financial systems—empowering you to stay ahead in an increasingly algorithm-driven market.
Table of Contents
The Rise of AI-Driven Portfolio Management
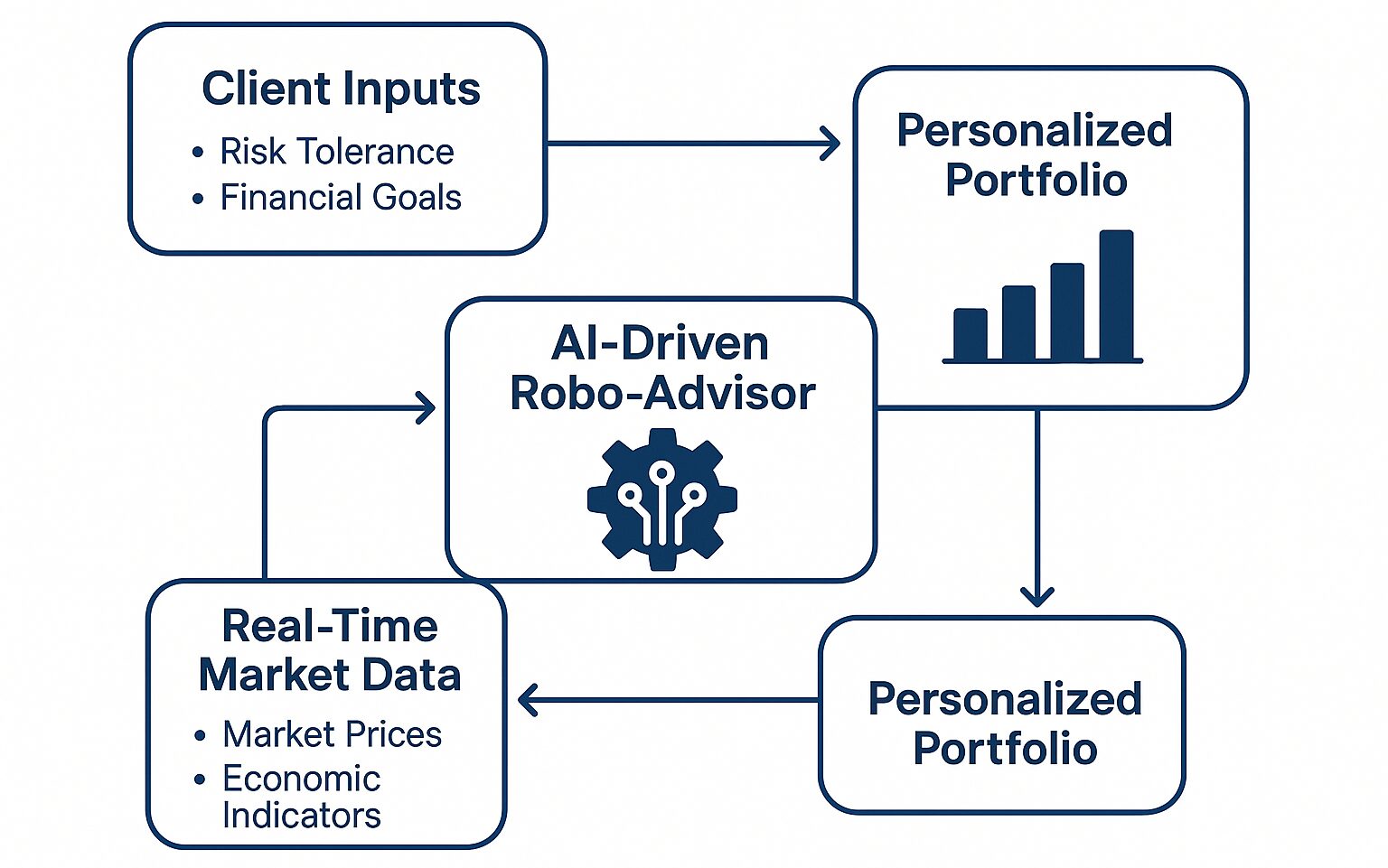
As technology continues to advance, the traditional methods of portfolio management—often characterized by manual analysis and delayed decision-making—are giving way to real-time, AI-driven processes. A new breed of robo-advisors powered by generative AI models has begun to dominate the market, providing personalized investment recommendations with unprecedented accuracy. Below, we explore how these AI-driven platforms are changing the game for investors.
Growth of Robo-Advisors
Robo-advisory platforms have evolved significantly over the last decade, moving beyond simple questionnaire-based approaches to sophisticated AI systems that can adapt to market changes in real-time.
- Generative AI and Real-Time Data: Modern robo-advisors increasingly rely on generative AI algorithms that process huge data sets—social media sentiment, macroeconomic indicators, earnings reports, and more—to make optimized investment decisions.
- Examples of Leading Platforms: Wealthfront and Betterment have been pioneers in this space, offering automated, diversified portfolios at relatively low fees. BlackRock, a global asset management giant, has also integrated AI-driven tools to enhance its portfolio construction and risk management processes.
Personalized Investing Strategies
One of the most powerful aspects of AI-driven portfolio management is the ability to create hyper-personalized strategies.
- Behavioral Data Analysis: AI tools can now interpret a wealth of behavioral data—like an individual’s spending habits, investment preferences, or even browsing history—to gauge risk tolerance and tailor portfolios accordingly.
- Goals and Time Horizons: By focusing on unique financial goals (e.g., retirement planning, home purchase, or short-term savings) and individual time horizons, these systems can dynamically shift asset allocations to align with changing life circumstances.
- Tax-Loss Harvesting: Many robo-advisors use machine learning algorithms to automatically scan for tax-loss harvesting opportunities, further optimizing an investor’s after-tax returns.
Benefits for Investors
AI-driven portfolio management opens up a range of benefits for both new and seasoned investors.
- Lower Fees: Automated platforms often cut out middlemen or reduce labor-intensive processes, leading to lower management fees.
- Accessibility: These tools are not limited to high-net-worth individuals. Retail investors can now access robust, data-driven strategies with just a few clicks.
- Real-Time Adjustments: Thanks to continuous data feeds, AI-driven systems can rebalance portfolios on-the-fly to capitalize on market volatility or protect against sudden downturns.
- Transparency and Education: Many AI-driven platforms also offer user-friendly dashboards that explain why certain trades were made, helping investors learn as they earn.
In summary, AI-driven portfolio management is transforming the investment landscape by making sophisticated strategies more accessible, personalized, and cost-effective. As these technologies continue to mature, expect an even greater degree of customization and performance optimization—elements that are essential for investors looking to stay ahead in the modern market.
Predictive AI for Market Sentiment & Macro Trends

Artificial intelligence has taken market analysis to a whole new level, particularly in the areas of sentiment tracking and macroeconomic trend forecasting. With the rise of Natural Language Processing (NLP) and Large Language Models (LLMs), AI can now sift through massive amounts of unstructured data—like social media posts, news articles, and corporate earnings calls—to gauge market sentiment and predict shifts in macroeconomic indicators.
The Power of NLP and LLMs
Modern NLP models and LLMs have become far more sophisticated at understanding context, tone, and even irony in written text.
- Sentiment Analysis: By applying advanced sentiment analysis to platforms like X, Reddit, and LinkedIn, AI tools can quickly detect shifts in investor sentiment surrounding specific stocks, sectors, or the overall market.
- Earnings Call Transcripts: AI-driven NLP engines can digest lengthy earnings call transcripts within seconds, extracting key phrases that might indicate bullish or bearish trends.
Tools and Applications
Leading financial institutions and tech companies are rapidly integrating these AI capabilities into their trading desks and analytical platforms.
- BloombergGPT: A prime example of a domain-specific LLM, BloombergGPT is trained on financial data to streamline the process of extracting insights from complex market reports and global news feeds.
- Sentiment AI Engines: Specialized sentiment AI engines offer real-time dashboards that correlate spikes in negative or positive sentiment with subsequent market movements. These engines can be especially valuable for day traders or high-frequency trading firms looking to capitalize on short-term volatility.
- Macro Trend Forecasting: Beyond social media sentiment, AI systems are also analyzing macroeconomic events—such as GDP reports, employment data, or central bank announcements—to forecast market performance. Some hedge funds integrate these forecasts into algorithmic trading models to gain a competitive edge.
Challenges and Considerations
While predictive AI for market sentiment and macro trends offers incredible promise, there are also challenges and ethical considerations.
- Data Quality: The accuracy of these models depends heavily on the quality and representativeness of the data. Biased or limited data sets can lead to skewed analyses.
- Interpretability: Many LLMs operate as “black boxes,” making it difficult for investors to understand exactly why the AI reached a particular conclusion.
- Regulatory Scrutiny: As AI models grow more central to market-moving decisions, regulators may demand greater transparency into the algorithms and the data sources they use.
By harnessing AI for real-time sentiment tracking and macro forecasting, investors can react more swiftly to emerging trends and gain a significant advantage. As the technology matures, we can expect to see even more granular insights—like sentiment breakdowns by geographic region, industry sector, and even the reliability of the data source.
AI in Risk Management & Fraud Detection
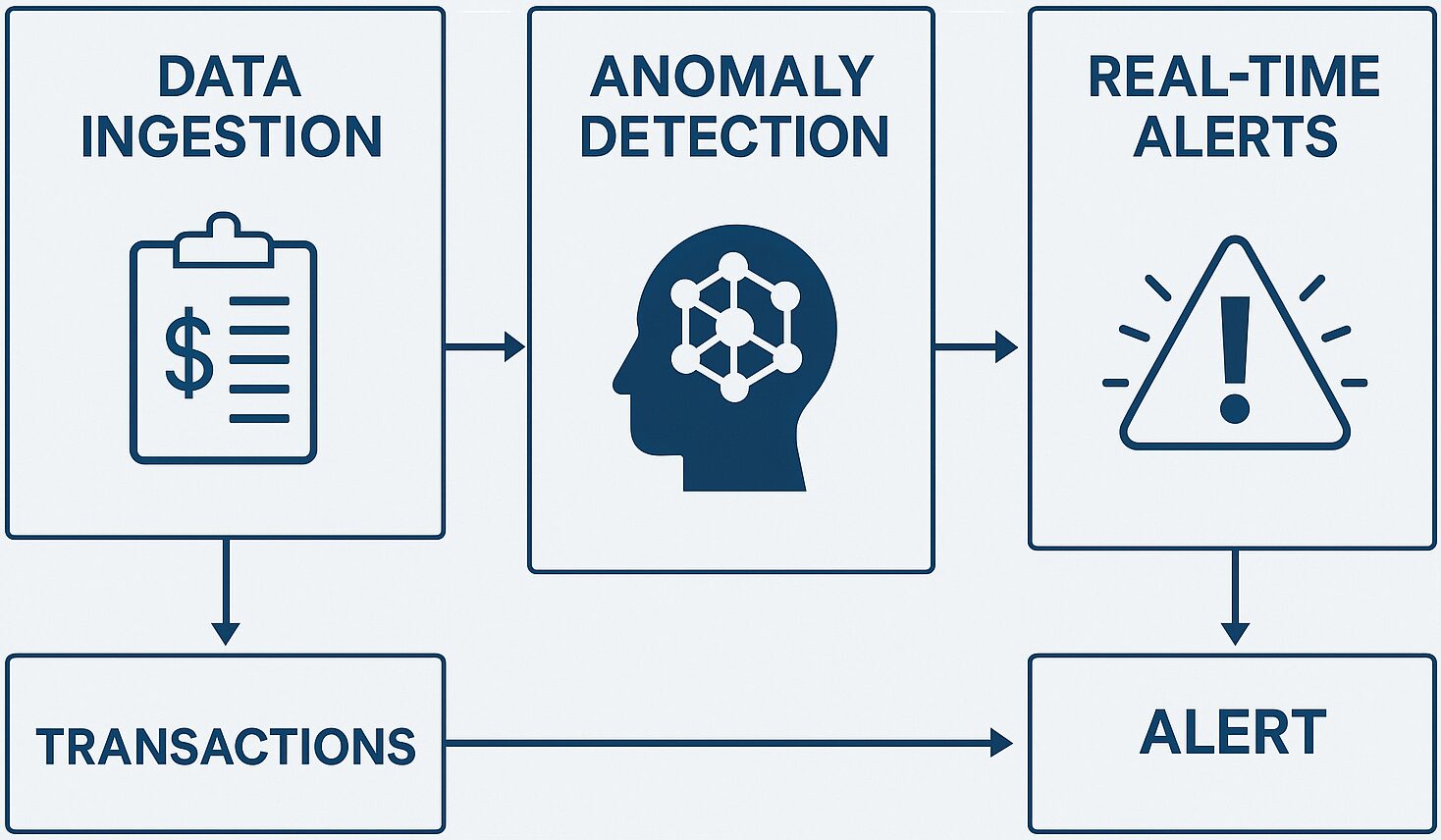
Risk management is at the core of finance, and AI-driven techniques are making it possible to identify and mitigate a range of threats in real-time. Beyond market risks, financial institutions also face the ongoing challenge of fraud—whether it’s payment fraud, identity theft, or sophisticated cyberattacks. AI models, particularly those utilizing machine learning (ML) and anomaly detection, are proving more adept than traditional methods in handling these multifaceted challenges.
Predicting Black Swan Events
One of the more ambitious applications of AI in risk management is the attempt to foresee low-probability, high-impact events, often referred to as “black swan” events.
- Anomaly Detection: Machine learning algorithms can flag unusual market activity and price movements by comparing them against historical norms. When combined with alternative data—like shipping data, weather patterns, and consumer trends—these models can detect early warning signs of market turbulence.
- Stress Testing: AI simulations can run thousands of stress-test scenarios with varying assumptions to see how portfolios, or entire financial systems, might behave in extreme conditions. This kind of testing goes beyond standard Value-at-Risk (VaR) calculations to uncover hidden vulnerabilities.
Real-Time Fraud Detection
Financial fraud has long been a thorn in the side of banks, payment systems, and trading platforms. Traditional rule-based systems rely on preset thresholds and patterns, often failing to catch new or rapidly evolving schemes.
- Adaptive ML Models: AI-driven fraud detection systems adapt to new fraud tactics by learning from fresh data. They don’t rely on rigid rule sets, thus offering a more flexible and robust solution.
- Behavioral Analysis: Modern fraud detection can include behavioral biometric data—like how a user typically navigates an app, the time of day they usually transact, or even keystroke patterns—to verify a user’s identity.
- Payment Gateways: Payment processors now deploy AI for immediate red-flagging of suspicious transactions, reducing the risk of large-scale fraud in real-time.
Enhanced Compliance and Risk Oversight
AI not only helps identify fraud but also aids in broader compliance-related risk management.
- RegTech Solutions: Automated compliance platforms use AI to scan vast amounts of transaction data for potential violations of Anti-Money Laundering (AML) or Know-Your-Customer (KYC) regulations.
- Real-Time Reporting: AI can produce near-instantaneous reports that regulators might require, drastically reducing the time and manpower needed for audits.
Balancing Privacy and Security
Although AI-driven systems provide robust security and risk management, they also raise questions about data privacy and the ethics of large-scale data collection.
- Data Minimization: Financial institutions are learning to apply privacy-by-design principles, limiting data usage to what is necessary for the specific ML tasks.
- Regulatory Compliance: Ongoing regulatory efforts, such as Europe’s General Data Protection Regulation (GDPR), constrain how and where data can be stored and processed.
By integrating AI into risk management and fraud detection, financial institutions stand a better chance of maintaining stability and trust in an increasingly fast-paced environment. From black swan event forecasting to real-time fraud detection, AI is delivering capabilities that were once thought to be out of reach.
Autonomous Trading Systems (AI Agents)
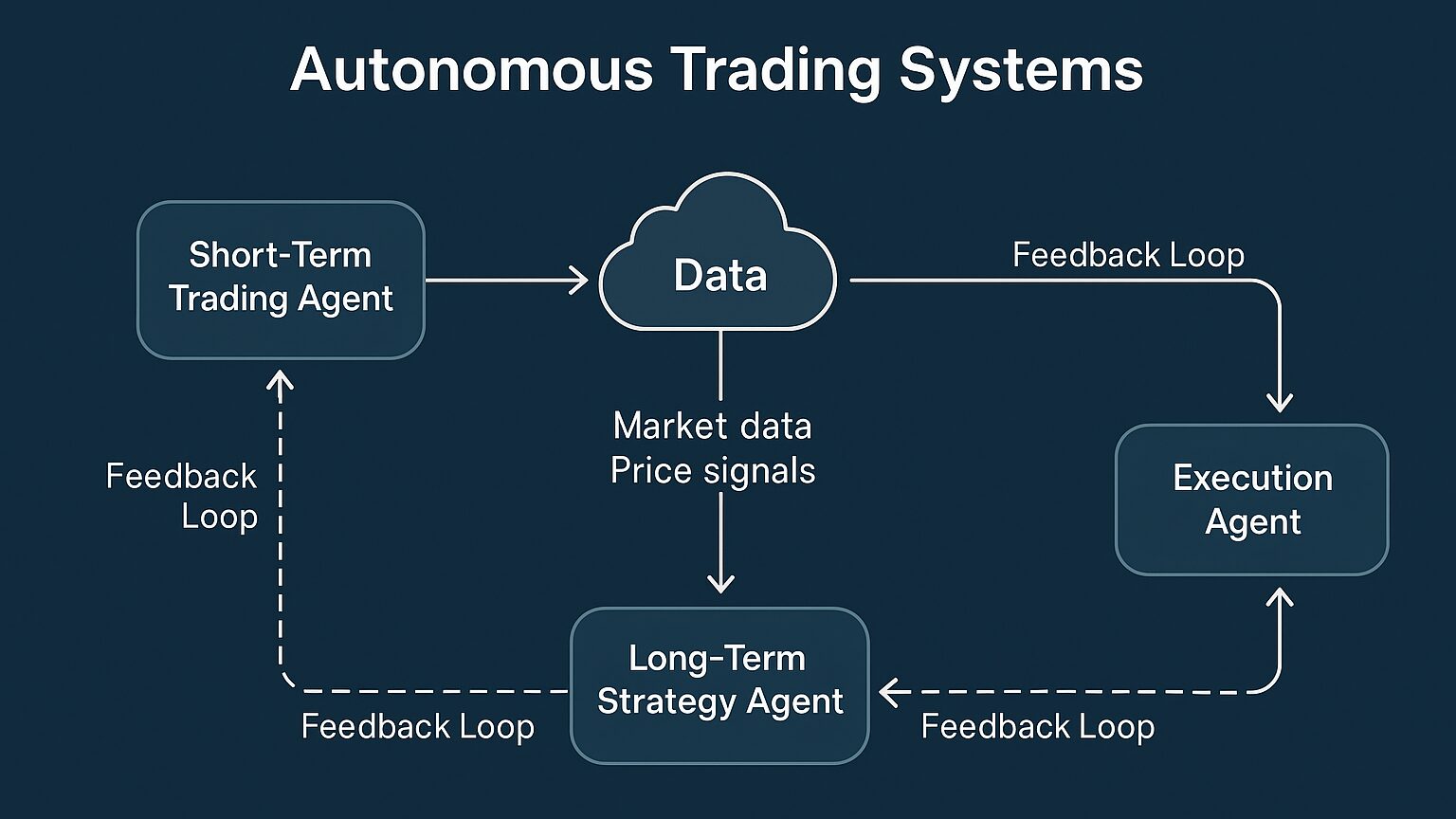
Autonomous trading systems—often referred to as AI agents—are reshaping the way trading desks operate by allowing algorithms to make and execute trades with minimal human intervention. These are not just simple formula-driven bots; they involve complex, multi-agent reinforcement learning strategies that continually adapt and improve based on real-world market feedback.
The Evolution of Algo Trading
Algorithmic trading has been around for decades, but the combination of big data, cloud computing, and advanced AI techniques has created a new generation of trading systems.
- Reinforcement Learning: Instead of merely following a preset algorithm, AI agents learn from market conditions over time, adjusting their models to maximize returns or minimize risk.
- Multiple Agents: In some systems, different AI agents specialize in different tasks—one might excel at short-term trades, while another focuses on long-term asset allocations. Over time, these agents interact, share insights, and refine each other’s models.
Continuous Improvement Loops
Modern autonomous trading platforms use continuous feedback loops to refine their strategies.
- Live Data Feeds: AI models ingest real-time market data—such as order book depth, trade volumes, and price changes—and immediately update their trading decisions.
- Cloud-Based Training: Training these models often requires massive computational resources. Cloud infrastructures offer the elasticity to scale up or down, enabling faster and more frequent retraining cycles.
- Performance Metrics: Regular evaluations measure both profitability and risk-adjusted returns, encouraging agents to find a balance between aggressiveness and caution.
Risk Mitigation and Controls
While fully autonomous systems can be highly effective, unrestrained AI-driven trading also carries the risk of exacerbating market volatility.
- Circuit Breakers: Many AI trading platforms incorporate circuit breakers that halt trading if performance falls outside a set range or if extreme volatility is detected.
- Human Oversight: Even in the most advanced autonomous setups, human portfolio managers or risk officers often remain in the loop to intervene in unexpected situations.
Ethical and Regulatory Dimensions
The autonomy of AI agents in trading raises new ethical and regulatory questions.
- Market Manipulation: Some fear that AI-driven trading could unintentionally manipulate markets if agents act in concert or exploit market inefficiencies too aggressively.
- Transparency Requirements: Regulators may demand more transparent algorithms or stress-testing procedures to ensure that these systems don’t introduce systemic risks.
Despite these challenges, autonomous trading systems offer a glimpse into the future of finance—where advanced AI collaborates with humans to drive efficiency, speed, and potentially higher returns. For investors, understanding how these systems function can be crucial, especially as they become a more integral part of the market ecosystem.
AI in Crypto & DeFi Risk Modeling
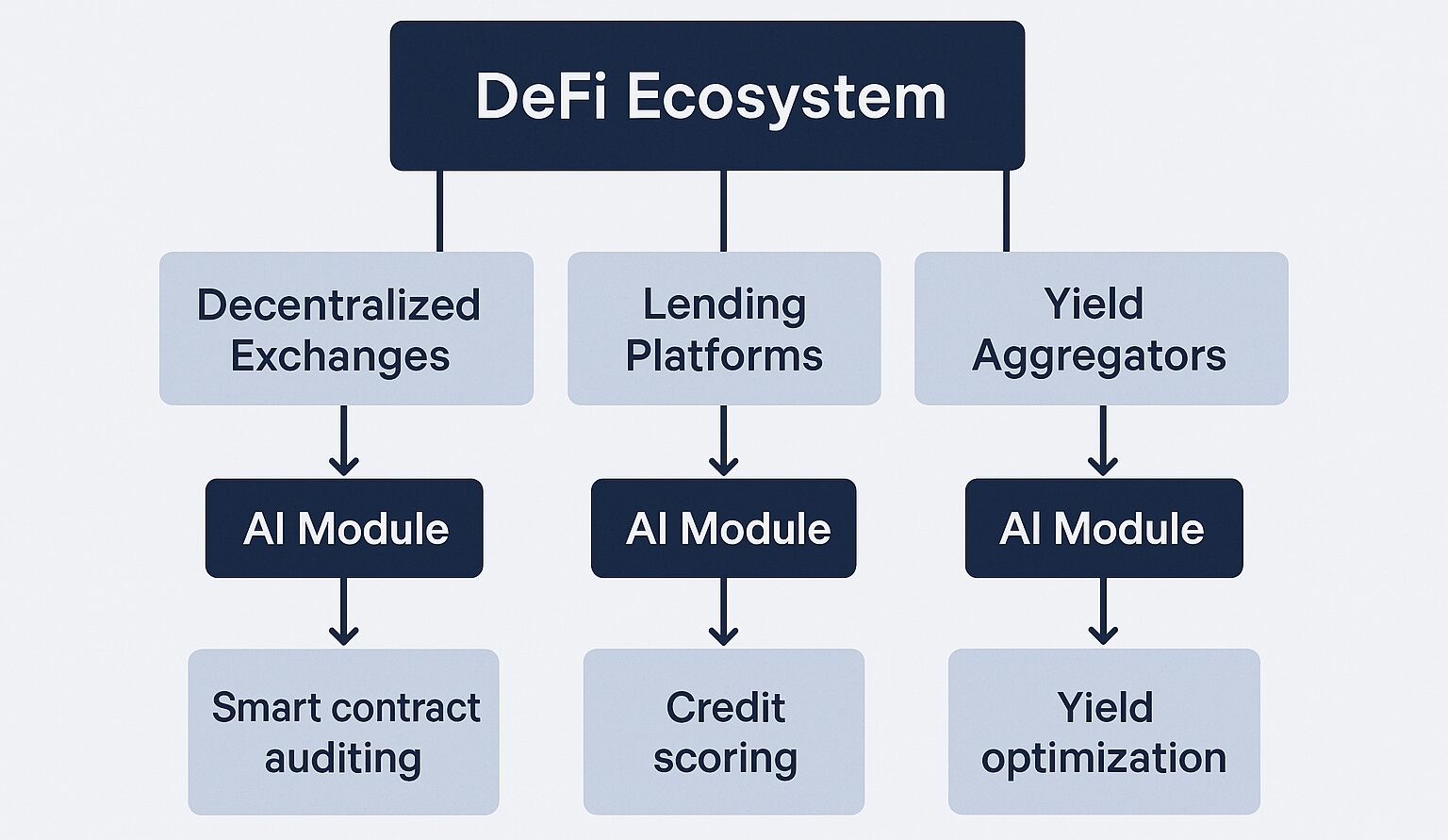
The cryptocurrency market and the broader Decentralized Finance (DeFi) space have matured dramatically in recent years. With this maturation comes greater complexity—thousands of tokens, smart contracts, yield-farming protocols, and on-chain metrics that even seasoned crypto enthusiasts find challenging to navigate. AI-driven solutions are stepping in to help manage this complexity.
Smart Contract Risk Analysis
Smart contracts lie at the core of the DeFi ecosystem, but bugs or vulnerabilities can lead to substantial financial losses.
- Automated Code Audits: AI tools can parse and analyze smart contract code for common vulnerabilities such as re-entrancy attacks, overflow errors, or logical flaws.
- LLM-Based Interpreters: Emerging large language models can read and summarize complex smart contracts in plain English, highlighting key terms, potential risks, and the implications for investors.
DeFi Yield Optimization
DeFi offers numerous yield-generation strategies, from liquidity provision to staking and lending. Yet, these strategies often involve intricate calculations and constant monitoring.
- Dynamic Allocation: AI-driven platforms automatically redistribute capital among different DeFi protocols based on real-time market conditions—interest rates, token prices, and liquidity metrics—to maximize returns.
- Risk-Adjusted Returns: Rather than just chasing the highest yield, sophisticated AI models weigh potential rewards against associated risks, like impermanent loss or contract exploits.
On-Chain Data Insights and AI Oracles
On-chain data—such as transaction volumes, wallet addresses, and protocol metrics—offers a trove of information for gauging market health and sentiment.
- AI Oracles: Traditional oracles like Chainlink feed external data into smart contracts. Next-generation AI oracles add an interpretive layer, filtering out anomalies and providing sentiment analyses.
- Predictive Analytics: By correlating on-chain metrics with external factors (e.g., social media sentiment or macroeconomic data), AI-driven systems can forecast potential price movements or liquidity crunches.
The Role of AI in Regulatory Compliance for Crypto
Regulation in the crypto space is still evolving, and AI can help projects and investors stay compliant.
- AML/KYC in DeFi: Some DeFi platforms are integrating AI-based tools for identity verification and transaction monitoring, aiming to preempt regulatory crackdowns.
- Regulatory Reporting: AI can compile transaction histories and risk metrics for regulators or auditors, streamlining compliance for crypto exchanges and DeFi protocols.
As we look to 2025, AI’s influence in the crypto and DeFi sectors will likely grow, helping investors make more informed decisions and minimize the unique risks inherent to digital assets. Whether it’s through smart contract analysis, yield optimization, or advanced on-chain analytics, AI will be central to the evolution of decentralized finance.
Human-AI Collaboration in Investment Strategy
Key Feature | Fully Automated System | Human-AI Collaboration |
---|---|---|
Decision Speed | Very fast decisions, real-time execution | Fast but reviewed by humans |
Interpretability | Low; decisions often a black box | High; humans can interpret AI outputs |
Adaptability | High adaptability with retraining | Moderate adaptability with human input |
Ethical Oversight | Limited ethical awareness | High ethical awareness via human oversight |
Cost Efficiency | Highly cost-efficient once built | Moderate, due to human resource involvement |
Bias Mitigation | Depends on training data quality | Better bias detection with human feedback |
Human Intuition | Lacks human reasoning | Strong human reasoning included |
Transparency | Opaque decision-making | Transparent and accountable |
Creativity | Not inherently creative | Allows human innovation and out-of-box thinking |
While fully automated systems garner significant attention, many experts believe that the future of finance lies in a hybrid approach—where humans and AI work together to make smarter investment decisions. This collaborative model is known as “AI-augmented investing,” a strategy that combines human judgment and intuition with AI’s ability to analyze massive data sets.
Why a Collaborative Approach?
AI models excel at pattern recognition, data mining, and speed, but humans bring essential qualities like intuition, creativity, and ethical reasoning.
- Reducing Behavioral Bias: Investors often fall prey to emotional biases—such as fear or greed—that can hinder rational decision-making. AI tools can provide objective analyses, tempering human biases.
- Expert Oversight: Portfolio managers can cross-check AI-driven insights against fundamental research or long-term economic indicators, ensuring that the final decisions align with broader investment objectives.
Co-Pilot Investing Platforms
Inspired by tools like GitHub Copilot (which assists programmers by suggesting lines of code), new fintech platforms offer “co-pilot” features for traders and analysts.
- AI Suggestions, Human Approval: Rather than executing trades automatically, the system presents suggestions—like “buy XYZ stock due to positive earnings revisions”—while leaving the final decision to the human operator.
- Natural Language Queries: Advanced LLMs allow analysts to ask natural language questions (e.g., “What are the top three performing tech stocks under $50?”) and receive immediate, data-backed answers.
Training and Skill Development
As AI takes on more complex tasks, financial professionals are compelled to upskill in areas like data science, machine learning, and programming.
- Hybrid Skill Sets: The ideal portfolio manager of the future might have both an MBA and a certificate in AI/ML, bridging the gap between quantitative and fundamental analyses.
- Educational Resources: Many online learning platforms and financial firms now offer specialized courses in AI for finance, helping current employees and students prepare for the hybrid roles that are becoming increasingly common.
Advantages for Retail Investors
Human-AI collaboration isn’t just for institutional investors. Retail investors also benefit from AI-augmented platforms that clarify investment decisions.
- Enhanced Understanding: These platforms often include explanatory notes, charts, and simulations, fostering financial literacy.
- Community Features: AI-powered “social trading” platforms allow users to follow or replicate the strategies of experienced investors, creating a community-driven approach to portfolio management.
By combining the strengths of both humans and AI, this collaboration aims to deliver more nuanced and robust investment outcomes. Rather than replacing human analysts, AI acts as a powerful tool, providing actionable insights quickly, enabling financial professionals and individual investors alike to navigate complex markets with greater confidence.
Regulatory AI and Compliance Automation
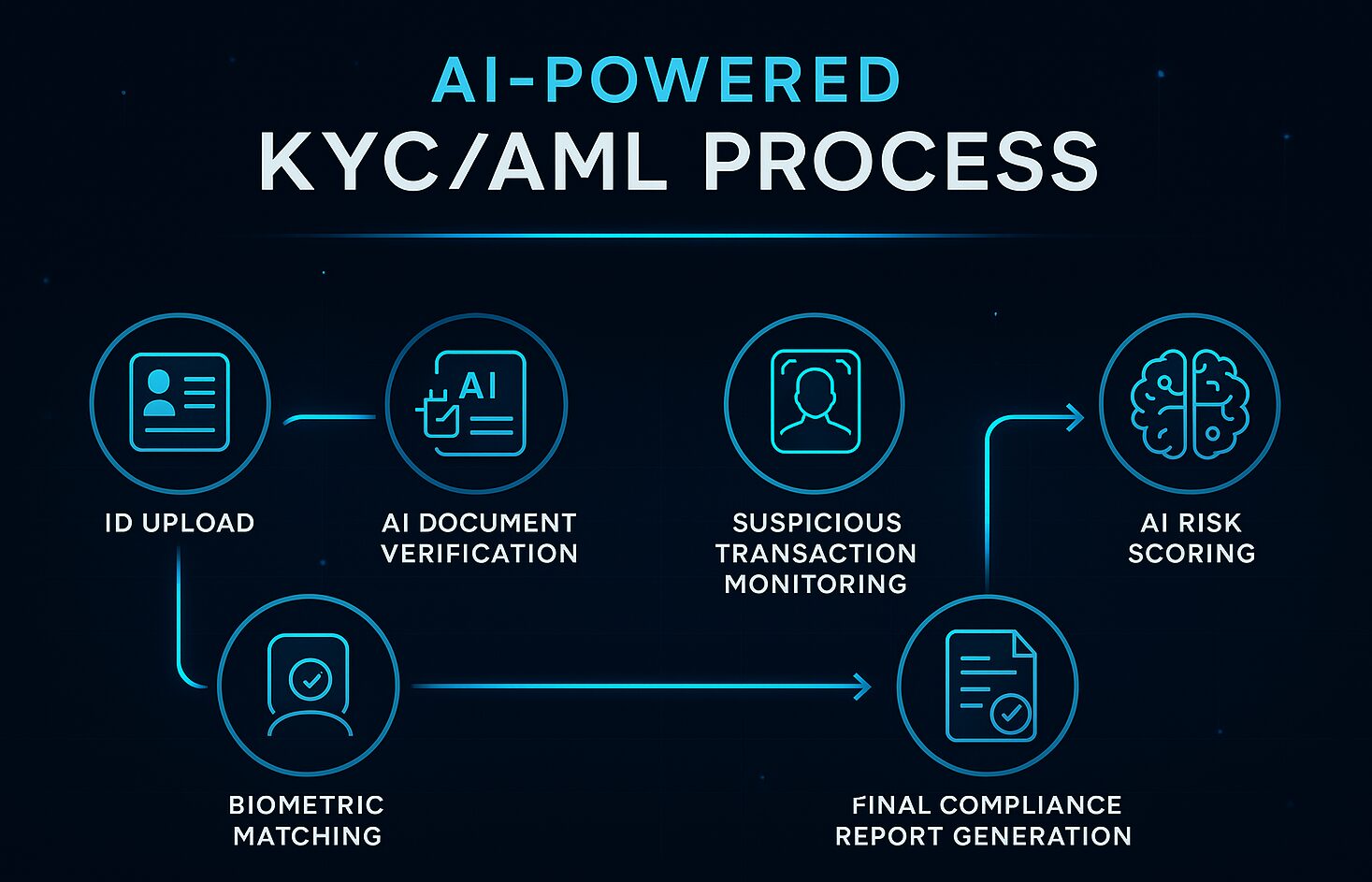
Financial markets are heavily regulated, and compliance requirements are ever-evolving. AI is emerging as a vital resource for maintaining compliance, reducing administrative overhead, and ensuring ethical conduct in an industry where missteps can be extremely costly.
Automating KYC/AML Processes
Know-Your-Customer (KYC) and Anti-Money Laundering (AML) measures are standard across the financial world.
- Identity Verification: AI can quickly verify documents like passports or driver’s licenses, checking for signs of tampering or fraud.
- Transaction Monitoring: Machine learning models detect patterns in transaction data that might indicate money laundering or other illicit activities. This can include abnormal transaction sizes, unusual time patterns, or suspicious geographic locations.
Regulatory Reporting and Audit Trails
Generating comprehensive audit trails and regulatory reports is often time-consuming and labor-intensive.
- Real-Time Updates: AI-driven platforms can compile relevant data throughout the day, significantly reducing the workload at the end of reporting periods.
- Automated Documentation: Large language models can summarize emails, trading logs, and other documents into easy-to-review formats for regulators or internal compliance teams.
Emerging Regulatory Frameworks
Governments worldwide are grappling with how best to regulate AI in finance.
- EU’s AI Act: The European Union is drafting legislation that could enforce strict risk classifications for AI systems. Financial AI tools might fall into higher-risk categories, requiring more rigorous assessments.
- Regulatory Sandboxes: Some jurisdictions are offering controlled environments where fintech startups can test AI-driven solutions under relaxed regulations, encouraging innovation while maintaining oversight.
Ethical AI and Bias Mitigation
As regulators and consumers become more aware of AI’s potential for bias, ethical considerations are growing in importance.
- Bias Audits: Firms increasingly conduct periodic bias audits of their AI systems to ensure that decisions regarding loan approvals, insurance underwriting, or credit scoring are not unjustly discriminating against certain groups.
- Explainability Requirements: Regulators may soon demand that AI models used in financial decision-making be able to provide human-readable explanations for their conclusions.
By automating compliance tasks and offering real-time oversight, AI reduces the risk of costly fines and reputational damage. At the same time, it enables financial organizations to remain agile and efficient in navigating complex regulatory landscapes.
Sustainability & ESG Screening via AI
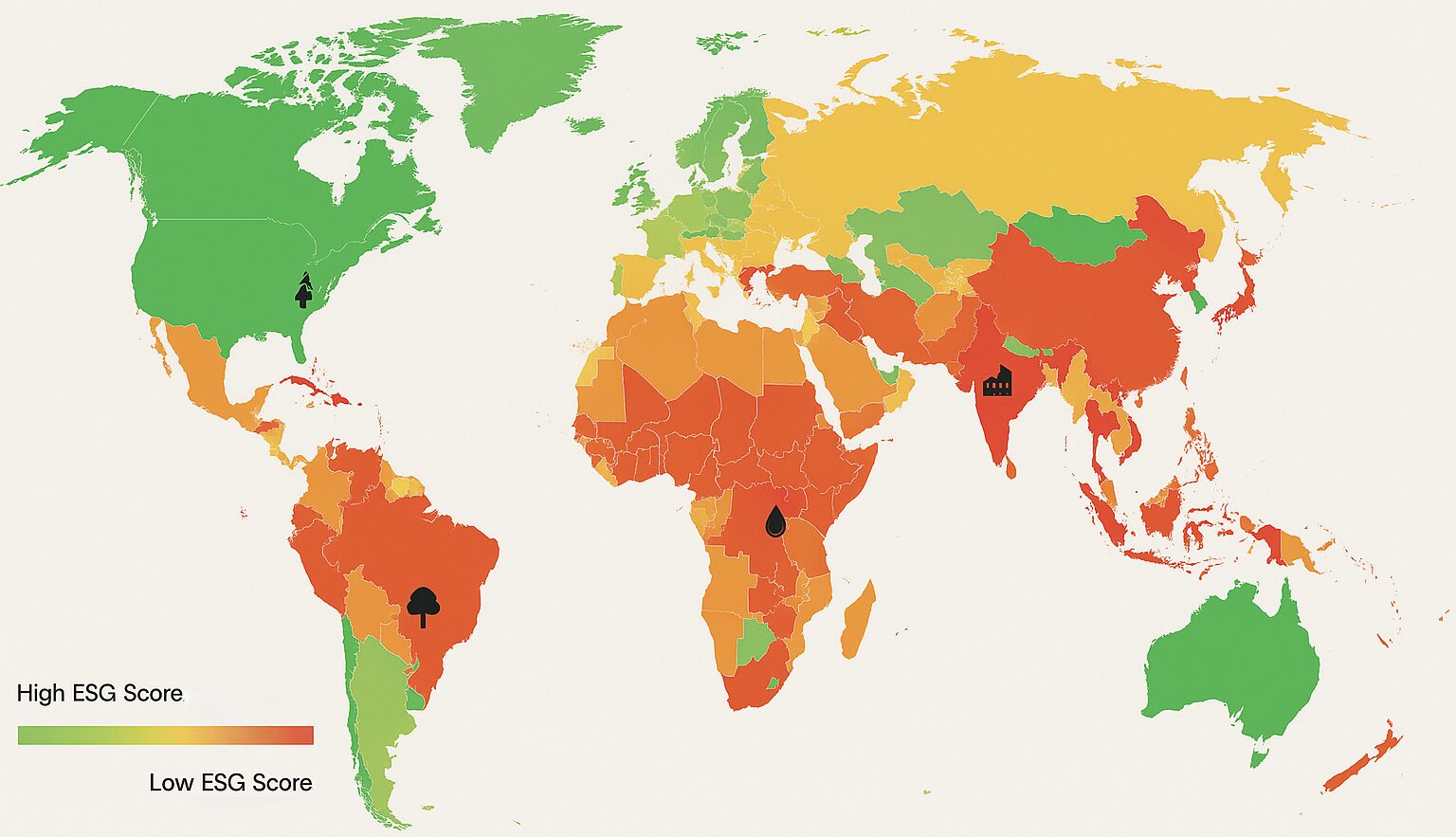
Environmental, Social, and Governance (ESG) investing has grown into a multi-trillion-dollar market. Institutional and retail investors alike are increasingly factoring sustainability metrics into their portfolios. AI is playing a pivotal role in aggregating and analyzing the diverse, and often unstructured, data necessary to evaluate a company’s ESG performance accurately.
Data Collection and Integration
ESG data can come from satellite imagery, corporate sustainability reports, supply chain records, and even sentiment analysis of a company’s brand perception.
- Satellite Data: AI can analyze satellite imagery to track deforestation, pollution levels, or changes in land use around industrial sites.
- Supply Chain Tracing: By mapping out global supply chains, AI can identify potential bottlenecks or high-risk regions, ensuring that raw materials are sourced ethically and responsibly.
Sentiment Analysis for ESG
Just as AI can assess market sentiment, it can also gauge public sentiment about a company’s social or environmental practices.
- Social Media Monitoring: Spikes in negative hashtags or coverage could signal ESG risks—like poor labor conditions or controversies over land usage.
- News Feed Analysis: AI models scan news outlets for mentions of environmental incidents or governance issues and correlate them with stock performance or brand reputation.
Climate Risk Modeling
Global climate change introduces new risks, from extreme weather events to supply chain disruptions.
- Scenario Planning: AI-driven climate models can forecast potential impacts on crop yields, energy consumption, and infrastructure damage. Investors use these insights to adjust portfolios accordingly.
- Insurance Industry Applications: Insurance companies increasingly rely on AI to model flood risks, hurricane paths, and other climate-related events, which in turn affects underwriting and premium calculations.
Mainstreaming ESG in Investment Strategies
ESG considerations are no longer niche; they’re becoming integral to risk management and value creation.
- Institutional Adoption: Large asset managers, including BlackRock and Vanguard, have signaled a strong commitment to ESG principles. AI helps these firms filter thousands of companies, highlighting those with robust sustainability profiles.
- Regulatory Mandates: Authorities in various regions are requiring more transparent ESG disclosures, pushing more companies to adopt robust reporting frameworks.
AI’s ability to collate and interpret sprawling ESG data sets allows for deeper insights than ever before. As sustainability metrics become a standard factor in investment decisions, investors who leverage AI-driven ESG analysis are better positioned to align their portfolios with both ethical standards and strong long-term performance.
AI’s Role in Democratizing Finance
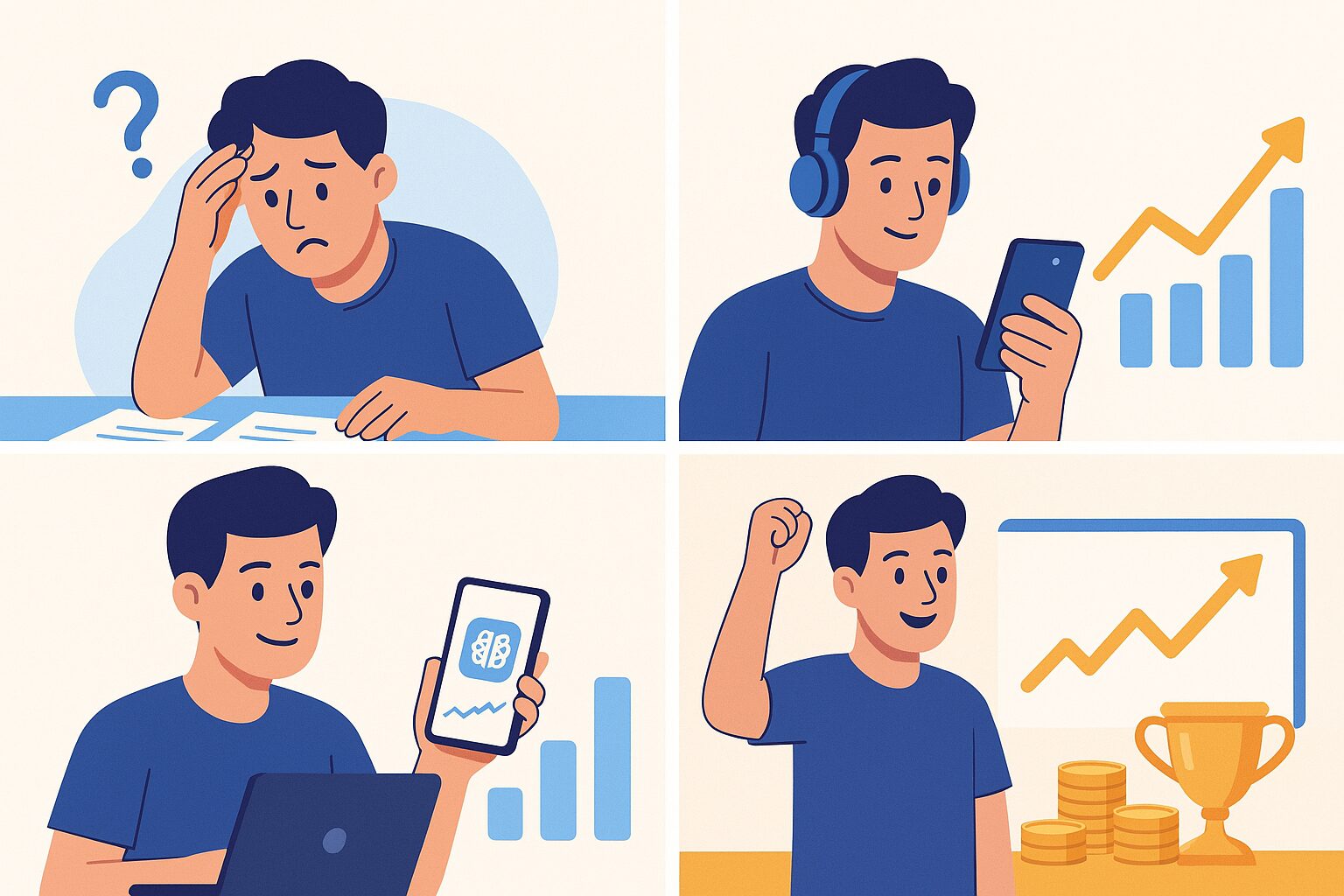
One of the most exciting developments in finance is the democratization of investment opportunities. AI-driven tools and platforms are making markets more accessible, transparent, and educational for a broader population—including those who have historically been underserved or intimidated by traditional finance.
Lower Barriers to Entry
High brokerage fees, complex trading interfaces, and a lack of financial literacy have been significant barriers. AI is changing that.
- Robo-Advisory Services: By offering low or no account minimums, robo-advisors enable small investors to partake in diversified strategies.
- Micro-Investing Apps: Many AI-enabled apps allow people to invest spare change or small recurring amounts, gradually building a portfolio without requiring large initial capital.
AI-Guided Financial Education
Education is critical for financial inclusion, and AI is making financial concepts easier to understand.
- Explainable Investing: AI-powered chatbots, often underpinned by large language models, can break down investment strategies in plain language, explaining complex terms like “compound interest” or “asset allocation” to beginners.
- Personalized Guidance: By analyzing a user’s behavior and financial data, AI-based educational platforms can recommend tailored lessons—teaching budget management, debt reduction, and basic investment principles.
Community and Social Trading
A growing trend involves community-driven or “social” trading platforms where AI tools facilitate communal learning and shared strategies.
- Copy-Trading AI: Users can replicate the trades of expert or AI-recommended portfolios. Over time, AI can highlight discrepancies and suggest improvements to a user’s strategy.
- Mentorship Programs: Some platforms link beginners with more experienced investors, with AI moderating the conversation to ensure reliable advice is shared.
Future Outlook for Inclusive Finance
As AI tools become more advanced and widespread, we can expect an even more profound democratization of finance.
- Beyond Borders: Fintech startups are exploring cross-border micro-lending platforms that utilize AI to assess creditworthiness, opening up credit lines to individuals in emerging markets who lack traditional financial histories.
- Women and Minority Investors: AI-driven platforms often aim marketing and educational efforts at underrepresented groups in finance, promoting a more inclusive investment environment.
By significantly reducing costs, simplifying complex strategies, and providing personalized educational resources, AI is paving the way for a more inclusive financial system. Whether you’re a seasoned trader or a novice investor, AI-driven democratization ensures that the tools and knowledge needed to succeed are more within reach than ever before.
FinGPT and Domain-Specific LLMs
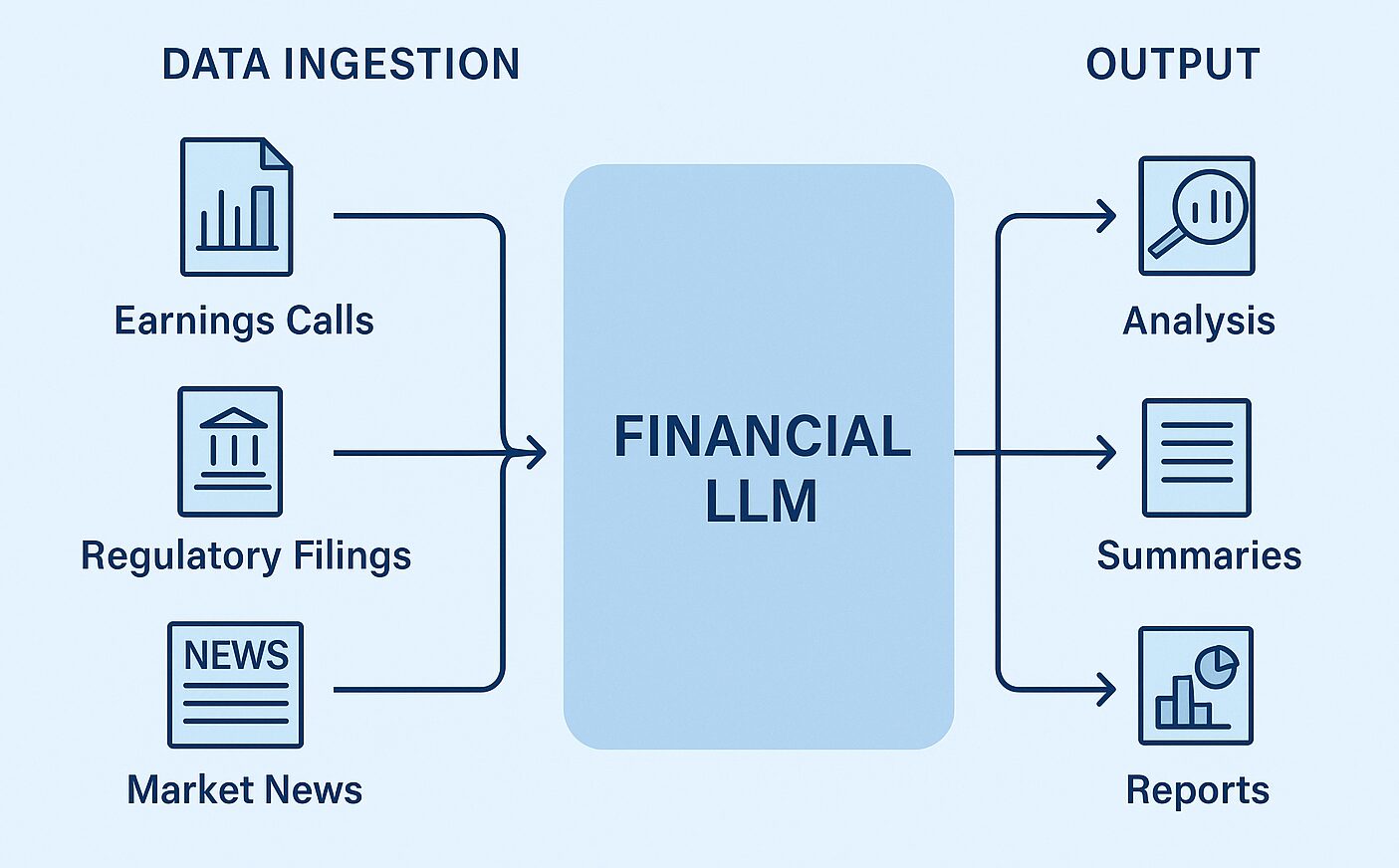
Large Language Models (LLMs) have gained prominence for their capacity to handle vast amounts of text and produce human-like responses. In finance, domain-specific models like BloombergGPT and open-source initiatives like FinGPT are at the forefront of this revolution, offering capabilities that surpass general-purpose models in terms of financial expertise.
What Are Financial LLMs?
Financial Large Language Models (FinLLMs) are specialized AI systems trained on data sets like earnings call transcripts, SEC filings, equity research reports, and market news.
- Domain Expertise: By focusing exclusively on financial texts, these models develop a more nuanced understanding of industry-specific terminology and context.
- Enhanced Accuracy: Compared to general-purpose LLMs, FinLLMs are less likely to produce irrelevant or erroneous answers when discussing specialized financial topics.
Key Players and Open-Source Initiatives
- BloombergGPT: Built by one of the largest data providers in finance, BloombergGPT caters to institutional clients by offering in-depth analysis and real-time insights.
- FinGPT: This open-source project aims to make financial LLM technology accessible to smaller firms, developers, and even individual enthusiasts.
- Private Hedge Fund Models: Hedge funds and proprietary trading shops often develop their own models in-house, leveraging confidential data to gain a competitive edge.
Applications of FinLLMs
Domain-specific LLMs offer a range of capabilities that can transform how analysts, traders, and executives operate.
- Automated Report Generation: By summarizing quarterly earnings or producing macroeconomic briefs, FinLLMs free up analysts for higher-level strategic tasks.
- Real-Time Financial News Interpretation: These models can quickly digest breaking news stories, corporate announcements, and regulatory changes, flagging those likely to impact specific assets or portfolios.
- Portfolio Intelligence: FinLLMs can help identify emerging market opportunities by cross-referencing multiple data sources—such as new patent filings, supply chain signals, or insider trading activity.
Challenges and Future Developments
- Data Privacy: Training large models often requires massive data sets, some of which may include proprietary or sensitive information.
- Ethical and Legal Risks: There’s a risk that these powerful models could be used to manipulate markets or engage in insider trading if misapplied.
- Technological Advances: Ongoing research in neural network architectures, reinforcement learning, and explainable AI could make FinLLMs even more powerful and transparent.
As these domain-specific LLMs refine their capabilities, investors and institutions that adopt them stand to gain a significant informational advantage. Whether it’s rapidly digesting corporate announcements or generating deeper insights into market trends, FinLLMs are set to become a cornerstone of modern financial analysis.
Conclusion
AI in Finance is redefining how markets operate in 2025—bridging the gap between Wall Street and everyday investors. From robo-advisors that deliver personalized strategies to LLMs analyzing real-time sentiment, AI is enhancing portfolio management, market insight, and fraud detection.
Hybrid trading systems now blend AI speed with human judgment, while in crypto and DeFi, AI supports smart contract auditing and on-chain analytics. Regulatory frameworks are evolving to ensure transparency, and ESG investing is being transformed by AI-powered climate and sentiment analysis.
Most importantly, finance is becoming more inclusive. Low-cost AI platforms and explainable investing tools are empowering retail investors like never before. With the rise of FinGPT and specialized financial models, the future belongs to those who embrace AI thoughtfully, stay informed, and adapt strategically in this new financial era.