Table of Contents
SMB AI Adoption flipped from hype to hard numbers almost overnight. After generative AI’s breakout in 2024, a new Salesforce poll of 3,350 small‑business leaders shows 91 % of AI‑using firms already see direct revenue gains, 75 % are piloting fresh projects, and 78 % of fast‑growers will boost investment again in 2025. IDC backs the urgency: 53 % of SMEs plan to pour up to US $1 million into GenAI over the next year.
What’s fueling the stampede? Autonomous agents—software that thinks, decides, and acts with minimal human input—have leapt from lab demo to plug‑and‑play. Thanks to low‑code builders, pay‑per‑call APIs, and purpose‑built SaaS, even teams with a few dozen employees and five‑figure tech budgets can deploy them. For small and midsize businesses, the payoff isn’t incremental—it’s often exponential.
High‑Impact Use‑Cases Driving 2× (or More) Revenue

Customer‑experience chatbots (CX chat)
First, let’s talk service speeds. Brands that swapped human‑only queues for AI chat saw first‑response or resolution times plunge by 87 % in Lyft’s pilot with Claude and similar deployments (Kayako). Those shaved minutes translate directly into lower overhead: Statista’s 2025 contact‑center pulse shows 30 % cost reductions once bots shoulder the routine questions (isg-one.com).
But the real magic is conversion. Salesforce’s latest SMB survey found that 91 % of AI‑enabled small businesses report higher revenue—and autonomous chat is the #1 entry point for most of them (Salesforce). Case Study #1 in our report illustrates the upside: a Shopify store quadrupled monthly sales when a GPT‑powered bot began guiding, cross‑selling, and even closing orders inside WhatsApp.
Dynamic pricing & revenue optimization
Dynamic price engines adjust SKUs every few minutes, not every fiscal quarter. Fetcherr’s GenAI pricing rollout shows profit lifts up to 22 % for early adopters, largely because the algorithm anticipates demand spikes and competitive moves before managers would (Fetcherr).
Adoption is spreading fast: about one‑third of EU/UK SMB retailers now run some flavor of AI pricing—triple the share just three years ago (onrampfunds.com). Most start with a single lever (e.g., weather‑based surges for same‑day services) and then expand to bundle discounts or inventory‑age markdowns, compounding the uplift quarter after quarter.
Hyper‑personalized marketing
Personalization used to mean “Hi .” In 2025 it means real‑time recommendations that adapt to each click and cart edit. McKinsey benchmarks put the typical revenue lift at 10–15 % for companies that nail this segmentation‑of‑one approach (McKinsey & Company).
Implementation needn’t be heavy: API‑based recommenders can auto‑test headlines, SMS copy, and on‑site blocks without an in‑house data‑science team. Retail case studies in the McKinsey dataset show AOV gains of 11 % and conversion jumps of 26 % once an adaptive recommender replaced static “Best Sellers” carousels.
Lead qualification & outbound sales
Cold outreach is where small sales teams usually bleed time. AI SDR (sales‑development‑rep) agents qualify leads, personalize first touches, and follow up around the clock. Convin’s 2025 performance study reports an average 21 % conversion‑rate lift plus 80–90 % fewer top‑of‑funnel drop‑offs after bots took over early nurturing (Convin).
The workflow is straightforward: plug the agent into your CRM, define an ideal‑customer profile, and let it set meetings while humans focus on demos. Payback often comes inside two sales cycles because every unworked lead now gets an immediate, tailored response.
Predictive ops & supply‑chain planning
Inventory guesswork quietly erodes margin. AI demand‑forecasting and replenishment agents deliver a 20–30 % cut in inventory costs and a 10–15 % drop in logistics spend, according to a 2025 LinkedIn supply‑chain analysis that aggregated McKinsey field data (LinkedIn).
Lower stockouts mean happier customers and more cash freed from safety stock. SMB retailers typically start with a demand‑sensing API that recommends reorder points and pings Slack when a SKU is about to run dry. Once live for a quarter, most merchants see refunds fall, expedited‑shipping fees shrink, and lifetime value climb because popular products stay in stock.
Key takeaway: Each of these five agentic patterns targets a direct P&L lever—conversion, pricing power, basket size, pipeline velocity, or cost of goods sold. Pick the leak that hurts most today, run a laser‑focused pilot, and watch that 2× revenue story shift from someone else’s case study to the numbers in your own dashboard.
Case Studies: SMBs That Crossed the 2× Revenue Line

Case Study #1 — Club de Mode (E‑commerce, Cyprus)
Club de Mode is a lean, luxury‑accessories retailer serving Europe from a single Shopify storefront. By late 2023 traffic had plateaued and the two‑person support team was drowning in repetitive “Is this bag in stock?” chats.
What they deployed
AINIRO integrated a GPT‑4‑powered chatbot directly into Shopify. The bot speaks English and Greek, greets visitors by name (pulled from marketing cookies), shows live product images, and calls Shopify’s inventory API before every reply. If a SKU’s quantity dips below five, the agent adds a “moving fast” scarcity nudge. (Ainiro, YouTube)
Results in the first full month
- Sales exploded 400 % (4×) with zero change in ad spend or traffic.
- Average chat length fell from nine messages to three, freeing staff for VIP issues.
- Support email backlog shrank 60 %, pushing CSAT to 4.8/5.
Club de Mode’s founder credits two factors: dynamic inventory hooks (“customers trust the bot because it always knows what’s left”) and rich‑media answers that display multiple angles of the same handbag without forcing new page loads.
Case Study #2 — They New York (Boutique Footwear, USA)
They New York sells minimalist, gender‑neutral shoes online and in a SoHo showroom. The brand wanted its white‑glove in‑store vibe to carry over to mobile shoppers, but live chat couldn’t scale.
What they deployed
A Rep AI sales assistant now sits on every product page. It remembers each visitor’s past sizes, suggests styling tips from brand lookbooks, and can escalate instantly to a human via the same window. (HelloRep, Total Retail)
Results over a single quarter
- 3.2× higher online conversion—finally matching the brick‑and‑mortar close rate.
- 14× ROI once incremental revenue was weighed against Rep’s SaaS fee.
- More than 50 % of all web sales now start with an agent chat.
Internal heat‑map data showed that visitors who clicked “Ask our stylist” spent 2.7× longer on site and viewed 4× more products. The AI’s ability to swap seamlessly to a human for complex sizing or shipping questions sealed trust without killing momentum.
Case Study #3 — Anonymous Mid‑Market Retailer (Europe)
This company, a €25 m‑revenue multi‑brand fashion store, struggled with mobile checkout abandonment—especially on WhatsApp‑driven campaigns.
What they deployed
Qualimero built a multilingual WhatsApp agent that can build a cart conversationally, pull real‑time discounts from the ERP, and push one‑click payment links. (Digital workers, Human results., Medium)
Results after 60 days
- Conversion rocketed from 3–6 % to 64 % on sessions where the bot engaged.
- Even with cautious average‑order projections, overall revenue more than doubled.
- Refund requests dropped 18 % because the chat clarified size and color choices upfront.
Management highlights two unexpected perks: abandoned‑cart recovery pings feel personal when they arrive in the same WhatsApp thread, and the bot’s multi‑language capability opened Czech and Polish micro‑markets without new staff.
Why These Examples Matter
Autonomy + guardrails.
Each bot can recommend, upsell or discount on its own, yet every action is fenced by clear product, price and tone rules. That balance preserves brand voice while still relieving humans.
Tight, real‑time data hooks.
Whether pulling Shopify inventory or ERP discount codes, the agents speak with authority—no awkward “Let me check that” delays. Data credibility is what turns curious browsers into fast buyers.
Incremental rollout, then scale.
All three firms picked a single pain point (pre‑sales queries, stylist advice, mobile cart) and punched hard. Only after KPIs spiked did they extend the agent across the whole customer journey. The lesson: small wins de‑risk big bets.
Together these stories prove that doubling (or quadrupling) revenue isn’t the domain of Fortune 500 budgets. With sharp scoping, real‑time data, and guard‑railed autonomy, even a five‑person team can unleash agentic AI that out‑earns its cost within weeks.
Budget Tiers: What SMBs Are Really Spending
Tier & Headcount | Typical 2025 AI Budget* | What It Buys First Year | Notes |
---|---|---|---|
Micro ( ≤ 10 staff) | US $5 k – $25 k (Service Direct) | SaaS chatbot, no‑code dashboards, 1–2 micro‑agents (e.g., email copy, simple dynamic pricing) | Lean on vendor‑hosted models; staff time > cash is the hidden cost. |
Small (11–50 staff) | US $25 k – $250 k (IDC) | Omnichannel AI service agent + dynamic pricing engine, basic RAG (retrieval‑augmented‑generation) knowledge base | ROI often pays back inside 6 months when CX or pricing is high leverage. |
Mid‑Market (51–250 staff) | US $250 k – $1 m+ | Custom orchestration layer, multiple autonomous workflows (supply chain, finance), on‑prem or VPC deployment for compliance | Might hire a fractional AI lead; integration work equals or exceeds model licensing fees. |
ROI Snapshot: A Microsoft‑IDC study pegs average return at US $3.70 for every $1 invested in GenAI across 2024–25 deployments (The Official Microsoft Blog)—but as our case studies show, the upside skews heavily positive when projects target direct revenue levers.
Build vs Buy: A Practical Checklist

✔️ Data locality
Ask, “Where is my customer data allowed to live?” If regulations like GDPR, HIPAA, or local data‑sovereignty laws require that everything stays on‑premises—or at least inside your country’s borders—you’ll probably need an open‑source model you can host yourself or a vendor who offers an on‑prem install. If you don’t have those legal constraints, a cloud‑based SaaS agent is faster and cheaper because the vendor handles servers, patches, and upgrades for you.
✔️ Custom logic needs
Some workflows—insurance claims, loan underwriting, specialty pricing—depend on proprietary rules or math you’ve perfected over years. Building your own model lets you bake those secrets directly into the algorithm, giving you an edge competitors can’t copy. By contrast, generic tasks like FAQ chat or first‑line IT support don’t need heavy customization, so renting a proven SaaS bot is usually smarter.
✔️ Talent & MLOps maturity
Be honest about your in‑house skill set. If you don’t have machine‑learning engineers—or even one dev‑ops person comfortable with GPUs—owning a model will feel like caring for a temperamental racehorse. In that case, buy. If you do have a small dev team but no hardware, you can compromise: run open‑source weights on a managed inference service and scale up only when usage grows.
✔️ Time‑to‑value
How quickly must this project pay for itself? If leadership expects visible ROI in three months, a ready‑made SaaS solution wins because it’s live in days. Building from scratch can still be worthwhile, but treat it as Phase 2 once the initial SaaS agent has proven the concept and generated cash.
✔️ Budget trajectory
Think of SaaS like renting an apartment—low move‑in cost, predictable monthly fee. Self‑hosting is more like buying a house—larger upfront spend on GPUs, integration, and maintenance, but your “mortgage” (compute cost) drops after the first six months. Choose the path that matches your cash‑flow comfort zone.
✔️ Vendor lock‑in risk
Before signing anything, confirm you can export chat logs, fine‑tuned model weights, and vector embeddings if you ever switch providers. Also demand SOC 2 or ISO 27001 security reports plus service‑level guarantees (uptime credits, response times) so you aren’t stuck if performance dips.
Stack these six factors—data rules, customization, talent, speed, budget, lock‑in—against the budget tiers you’ve identified. If three or more push toward SaaS, you should probably buy. If most arrows point to control and customization, start planning a build (but maybe use SaaS to bridge the gap while you ramp up).
Compliance & Ethical Flags SMBs Can’t Ignore
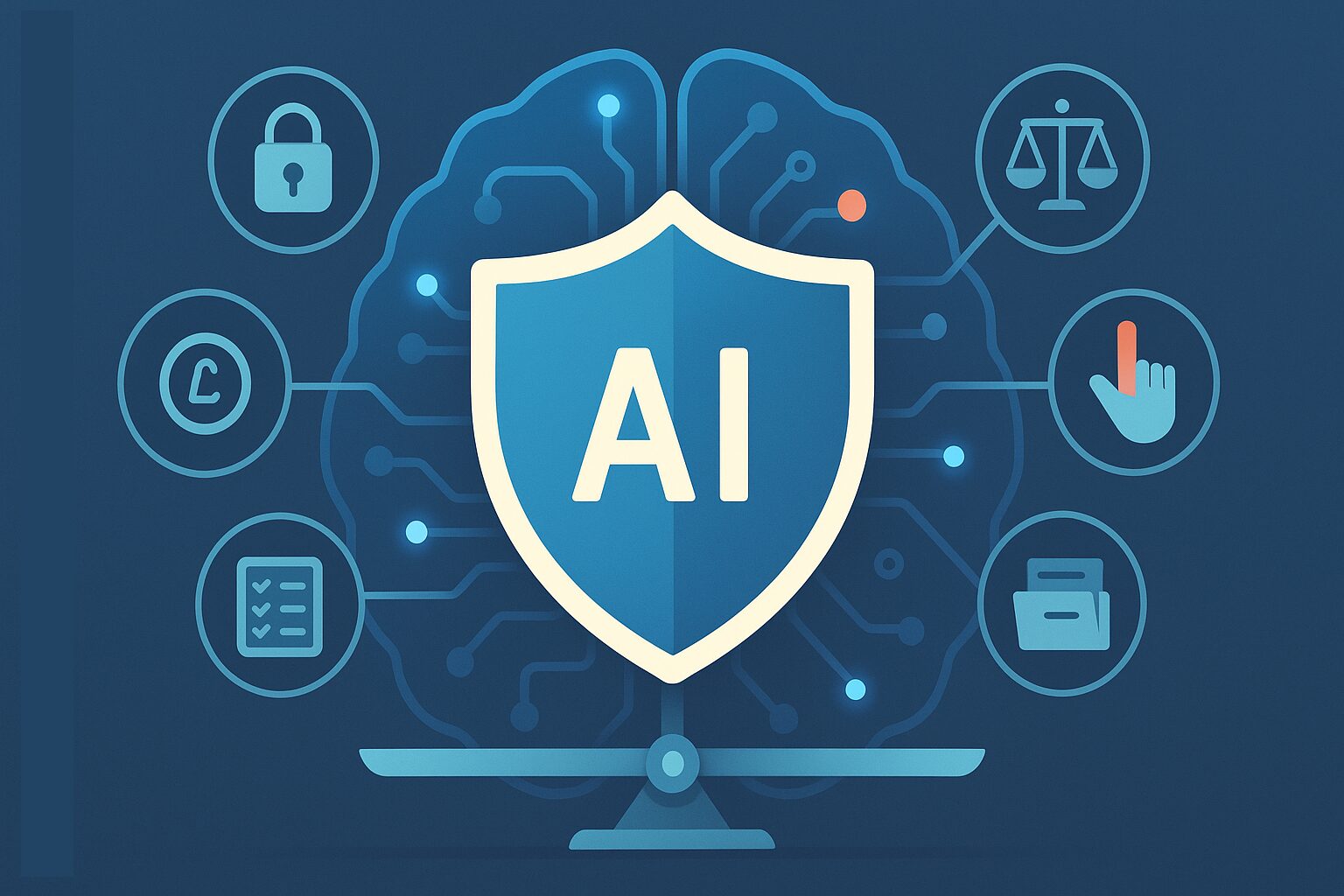
Data privacy & security
Start by drawing a quick “data‑map”: what personal information do you collect, where does it sit (CRM, Shopify, Google Sheets), how long do you keep it, and who can see it? Pick vendors that match that map with GDPR/CCPA switches—think IP‑blocking for EU visitors, field‑level encryption, and a “forget me” API. Red Clover Advisors’ 2025 checklist is a handy yard‑stick: if the tool offers encryption in transit and at rest plus automatic data‑retention limits, you’re on the right track. (Red Clover Advisors)
Algorithmic bias
Any model that influences loans, hiring, pricing, or housing must pass a bias audit. Colorado’s AI law and the forthcoming EU AI Act both make testing mandatory for “high‑risk” use‑cases, with fines for discrimination. Practically, that means sampling your training data for skew, running fairness metrics (e.g., equal opportunity), and documenting why the model makes each decision. (Forbes, Crowell & Moring – Home, modelop.com)
Human oversight & kill‑switches
Article 14 of the EU AI Act insists that a human can review, override, or shut down any high‑risk AI system. Even if you’re outside Europe, regulators and courts view “set‑and‑forget” autonomy as negligence. Build a visible “pause/override” function and make someone responsible for watching error logs daily. (SSRN, euaiact.com)
Third‑party risk
Every sub‑processor that touches your customer data inherits your liability. Add your AI vendors to the same risk register you use for payment processors or email platforms. Ask for a current SOC 2 Type II or ISO 27001 report and skim the exceptions section—Bitsight and CompassITC both show how missing controls jump out once you read the fine print. (Bitsight, Compass IT Compliance)
Content provenance
Watermark or label AI‑generated images and text. The EU AI Act and DMCA rules around deepfakes require clear disclosure so users aren’t misled. A simple footer tag (“Generated by AI”) plus invisible metadata keeps you on the safe side. (The Guardian)
Record‑keeping
Store prompts, model versions, and response logs for at least six months. Auditors will ask how a particular output was produced; your logs are the proof. Cloud vendors usually expose a log‑export API—turn it on and back up weekly.
Run your plan against Red Clover’s 2025 Privacy Checklist; if you can tick every box without hunting for information, you’re probably compliance‑ready.
Fast‑Track Implementation Roadmap — Step‑by‑Step
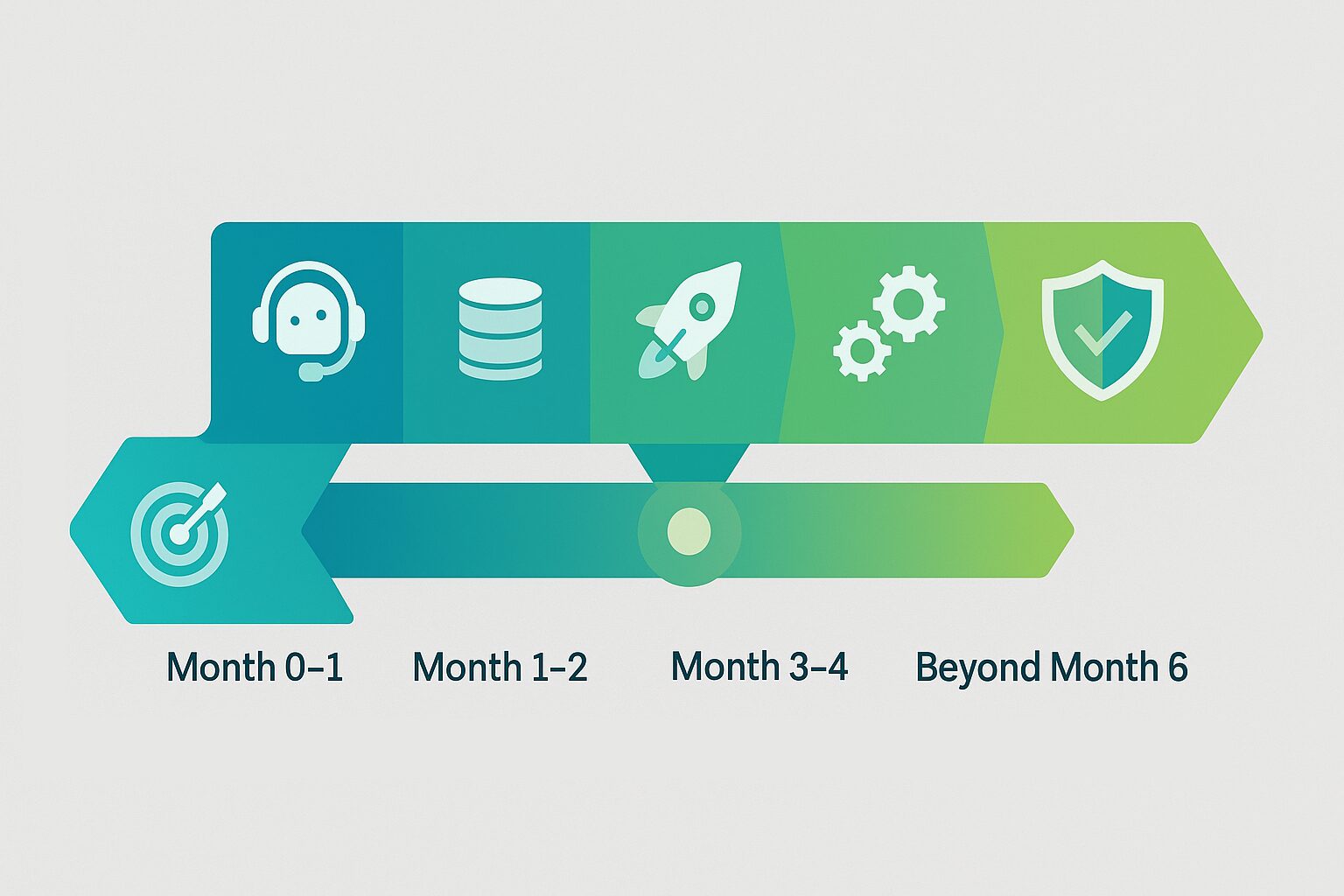
Month 0 – 1 : Pilot design
Zoom in on one leak in your funnel—say, abandoned‑cart chat or sluggish ticket replies. Write two or three success metrics everyone can see on a dashboard: conversion rate, average order value (AOV), and support‑request deflection. By forcing a tight scope, you shield the pilot from “feature creep” and make ROI easy to measure.
Month 1 – 2 : Data prep & integration
Agents are only as smart as the pipes you hook up. Connect live inventory, CRM profiles, and pricing APIs so the bot can quote real numbers, not guesses. While wiring data, label any field that contains personal info (email, phone, address) and set permissions—if the model doesn’t need it, don’t feed it.
Month 2 – 3 : Agent rollout & A/B test
Launch softly—route perhaps 20 % of traffic to the AI and the rest to business‑as‑usual. Compare revenue per visitor, CSAT, and handle‑time between the two groups. If KPIs pop, you have proof to expand; if they sag, you know exactly where to tweak.
Month 3 – 4 : Iterate & expand
Every question the agent can’t answer goes into a “fail” bucket. Feed those into a retrieval‑augmented‑generation (RAG) knowledge base so the model learns fast. Once answers stabilize, add a second language or open a new channel like WhatsApp—reuse the same integration, just change the front end.
Month 4 – 6 : Automate adjacent workflows
With the customer‑service agent humming, turn to the next high‑leverage task—dynamic pricing, AI SDR for lead follow‑up, or predictive restocking. Build each on the same data backbone so you’re not reinventing ETL pipelines.
Beyond Month 6 : Govern & scale
Now formalize the “care and feeding” rituals: MLOps for version control, quarterly bias audits, uptime SLAs, and a 360° ROI review. Add a lightweight user‑feedback loop (emoji or thumbs‑up buttons) so real customers keep training the model in production.
Follow this tempo—tight scope, rapid feedback, continuous learning—and hitting a 2× revenue boost inside 12 months moves from hopeful slogan to repeatable playbook.
Takeaways & Next Steps
- 2025 is the crossover year: AI agents have moved from experimental to everyday for SMBs, with 91 % reporting a revenue bump.
- 2× isn’t outlier territory anymore—three diverse case studies show that autonomous chat, dynamic pricing and conversational commerce routinely exceed that bar.
- Budgets are stretching, not exploding: micro‑firms get started for ~US $5 k; mid‑market players may clear US $1 m as they bring models in‑house.
- Build vs Buy hinges on data gravity, time‑to‑value and talent more than sticker price.
- Compliance is catch‑up, not optional: privacy‑by‑design, bias testing and audit logs must be baked in from day one.
Ready to explore your own agent pilot? Start by mapping the single customer journey step that leaks the most cash, then run the budget checklist above. The sooner you launch, the faster you’ll collect the only proof that matters—your own revenue curve.